Artificial intelligence (AI) is rapidly being adopted across the financial landscape. In asset management, applications of AI are articulated around the technology’s key inherent capabilities: the ability to conduct repetitive tasks efficiently; the ability to extract information from unstructured data sources; and the fact that AI algorithms are able to improve themselves, according to a research paper by the CFA Institute.
The paper, titled Artificial Intelligence in Asset Management, gives an overview of a wide range of existing and emerging applications of AI in asset management, focusing on three major areas: portfolio management, trading and portfolio risk management. Looking at how the technology has been used so far in the industry, it outlines three main intrinsic capabilities of AI that are relevant to asset management.
The first capability, the paper says, relies on the fact that AI models are objective, highly efficient in conducting repetitive tasks, and able to identify patterns in high dimensional data that may not be perceptible by humans.
AI can also analyze data with minimal knowledge of their structure or the relation between input and output, a feature that’s is especially useful for forecasting, yielding more accurate estimates, the paper says.
In trading, AI techniques are already an essential part of the practice, notably for its ability to process large amounts of data to generate trading signals. Moreover, algorithms can be trained to automatically execute trades based on these signals, which has given rise to the industry of algorithmic trading.
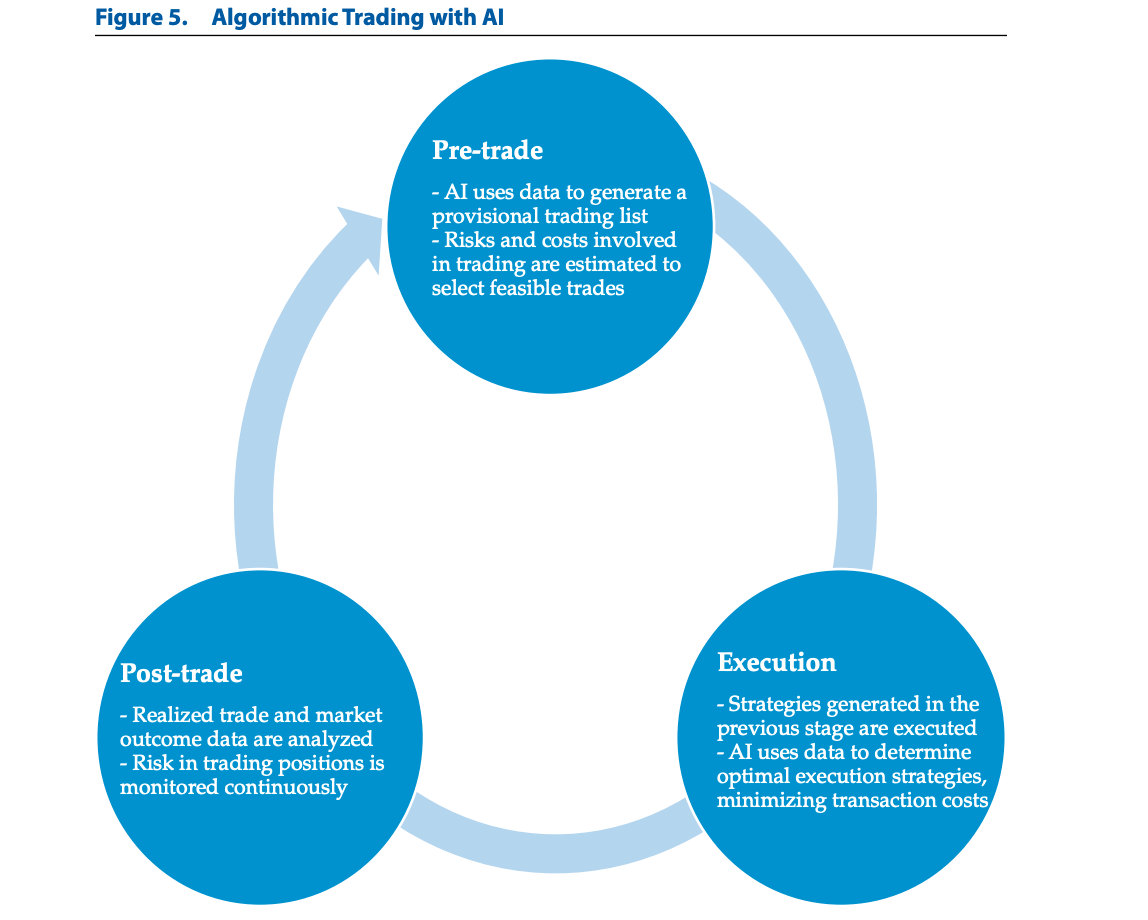
Note: The figure presents the three stages of algorithmic trading and summarizes the applications of AI in each stage.
In portfolio management, AI is being used to help construct portfolios with specific risk and return characteristics. AI has helped produce better risk estimates and solve portfolio optimization problems, thus improving the shortcomings of classical portfolio construction techniques and producing better asset return, the paper says.
The second capability, it notes, is the ability of AI to extract information from unstructured data sources, such as news articles, online posts, reports, and images. As a result, a tremendous amount of information can be incorporated into financial analysis without manual processing and intervention.
This is relevant for risk management where AI currently helps generate more accurate forecasts of bankruptcy and credit risk, market volatility, macroeconomic trends, financial crises, and so on, than traditional techniques.
Finally, the third capability outlined in the paper is the fact that unlike other statistical techniques, AI algorithms can be designed to improve themselves by readjusting in accordance with the data. This ability, it says, means that the manual reconfiguration or parameter re-estimation is unnecessary with AI.
Adoption of AI on the rise, though DACH lags behind North America, Asia
In recent years, AI have surged in popularity with machine learning (ML) techniques being the most used in finance right now, the paper says. ML is a subset of AI in which machines are able to decide and perform actions based on past experiences.
This surge in popularity has been mainly driven by three developments, the paper says: improved computing processing and storage capacity; increased volume and breadth of data used to train AI models; and greater accessibility and improvements of AI algorithms.
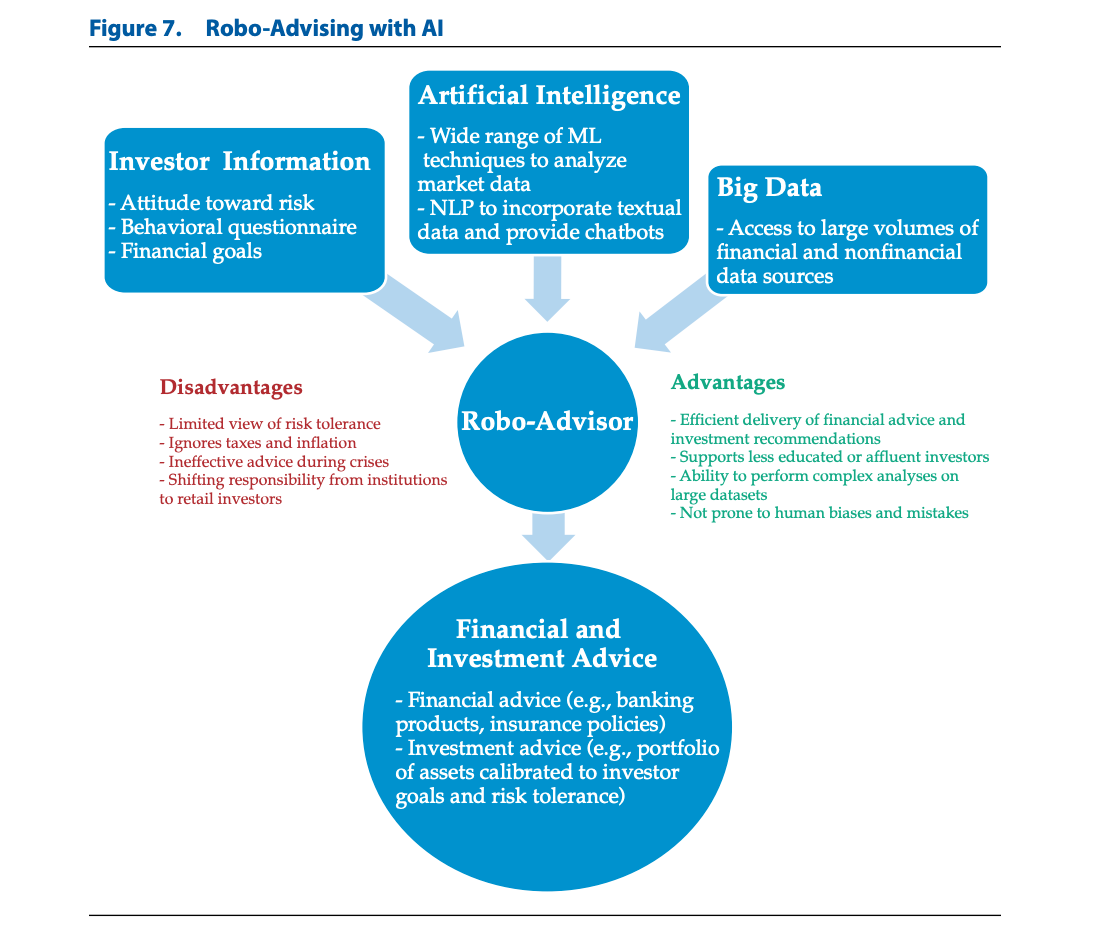
Note: The figure illustrates the structure of robo-advisor systems that incorporate AI and summarizes the advantages and disadvantages of these systems.
According to Insider Intelligence’s AI in Banking report, the decision for financing institutions to adopt AI will be accelerated by technological advancement, increased user acceptance and shifting regulatory frameworks.
But in the DACH region (Germany, Austria and Switzerland), AI adoption still lags behind more advanced regions like Asia and North America, according to a 2020 PwC study.
To date, DACH’s AI activities have been primarily focused on smaller-scale business and customer experience improvements. 56% of survey respondents, which consisted of banks, insurers and fintech professionals in DACH, said they were investigating AI for personalization and chatbot experiences, and an overwhelming majority (80%) said they were hoping to use AI for smaller efficiency improvements such as smaller-scale automation and predictive marketing.
AI applications could help banks save an estimated US$447 billion by 2023, with front and middle office accounting for US$416 billion of that amount, according to a research by Autonomous Next.
Featured image credit: edited from Science vector created by iuriimotov – www.freepik.com
The post 3 Key AI Capabilities for Asset Management Applications appeared first on Fintech Schweiz Digital Finance News - FintechNewsCH.
Comments